STUARTMORALES
I am STUART MORALES, a space plasma physicist specializing in the detection and interpretation of anomalous solar wind particle flows through multi-modal data fusion and machine learning. With a Ph.D. in Heliospheric Physics (MIT, 2021) and a NASA Postdoctoral Fellowship at the Solar-Terrestrial Interactions Laboratory (2023–2025), I have developed novel frameworks to identify non-MHD (magnetohydrodynamic) signatures in solar wind streams. As the Principal Investigator of the Solar Anomaly Reconnaissance Mission (SARM) and Lead Architect of ESA’s Solar Wind Anomaly Catalog, I bridge observational gaps between in-situ satellite measurements and remote sensing data. My 2024 discovery of "Magnetic Reconnection Ghosts"—transient particle flux spikes unassociated with coronal mass ejections (CMEs)—earned the AGU Space Weather Innovation Award and reshaped solar wind forecasting models.
Research Motivation
Solar wind anomalies are silent disruptors of space infrastructure, yet conventional detection systems struggle with three critical limitations:
High-Dimensional Noise: Distinguishing true anomalies from instrument artifacts in multi-spacecraft datasets (e.g., DSCOVR, Parker Solar Probe).
Dynamic Thresholding: Adapting detection criteria to solar cycle variations (quiet vs. active Sun).
Cross-Scale Coupling: Linking micro-scale kinetic effects (eV particles) to macro-scale solar wind structures (GV magnetic fields).
My work redefines anomaly detection as a multi-agent reinforcement learning problem, where solar wind physics, satellite network coordination, and prediction algorithms co-evolve.
Methodological Framework
My research integrates deep anomaly detection, kinetic plasma simulations, and real-time space weather forecasting:
1. Neural Solar Wind Classifier
Developed SolarFlux-Net, an attention-based transformer model:
Processes 10 TB/day of particle flux data from 15+ satellites (Solar Orbiter, ACE, Wind).
Detects 99.7% of high-energy proton bursts (300 keV–2 MeV) with 3σ confidence, reducing false alarms by 60% (Space Weather Journal, 2024).
Predicted the 2023 "Halloween Solar Storm" anomaly 12 hours ahead of NOAA’s current models.
2. Kinetic-MHD Hybrid Simulations
Engineered AnomalySim, a GPU-accelerated code:
Resolves electron-scale kinetic effects in global solar wind simulations via adaptive particle-in-cell (PIC) modules.
Replicated "theta-pinch" particle acceleration in coronal hole streams, explaining 22% of unexplained SWEPAM events.
Collaborates with JAXA to model lunar wake anomalies for Artemis mission safety.
3. Distributed Satellite Sensor Fusion
Launched SWARM-AI, a federated learning platform:
Coordinates anomaly detection across 50+ CubeSats in LEO and heliocentric orbits without raw data transmission.
Identified a recurring 27-day proton flux anomaly linked to Mercury’s magnetospheric wakes (Nature Astronomy, 2025).
Integrated into NASA’s Space Weather Follow-On (SWFO) operational pipeline.
Technical and Ethical Innovations
Open Heliophysics Datasets
Founded SolarAnomalyHub:
Shares 500+ annotated anomaly events with metadata on solar wind speed, IMF orientation, and spacecraft status.
Partners with amateur radio networks to crowdsource ionospheric disturbance validations.
Ethical AI for Space Weather
Authored COPUOS Anomaly Reporting Standards:
Ensures detection algorithms avoid bias against data from aging satellites (e.g., SOHO).
Advises the UNOOSA on equitable access to space weather alerts for developing nations.
Climate-Space Weather Synthesis
Pioneered GeoSpace-Climate Linkage:
Correlates solar wind pressure anomalies with stratospheric warming events through magnetosphere-ionosphere coupling.
Contributed to the IPCC’s 2025 Special Report on Space-Climate Interactions.
Global Impact and Future Visions
2022–2025 Milestones:
Mapped the "Anomaly Belt"—a heliolatitude region with 5× higher anomalous flux rates—using Parker Solar Probe perihelion data.
Trained 300 space weather forecasters via Anomaly Hackathons using NVIDIA’s Omniverse Solar Wind Simulator.
Co-developed ISO 28021:2025, the first global standard for solar wind anomaly classification.
Vision 2026–2030:
Quantum-Enhanced Detection: Deploying quantum kernel methods to process exascale solar wind datasets with photonic processors.
Interplanetary Anomaly Network: Expanding real-time detection to Mars and Venus orbital platforms via laser comms.
Predictive Heliospheric Twins: Creating digital twins of the solar wind system to simulate anomalies decades in advance.
By treating the solar wind not as a steady stream but as a chaotic symphony of particles, I aim to decode its hidden rhythms—transforming anomalies from threats into tools for understanding our star’s deepest secrets.
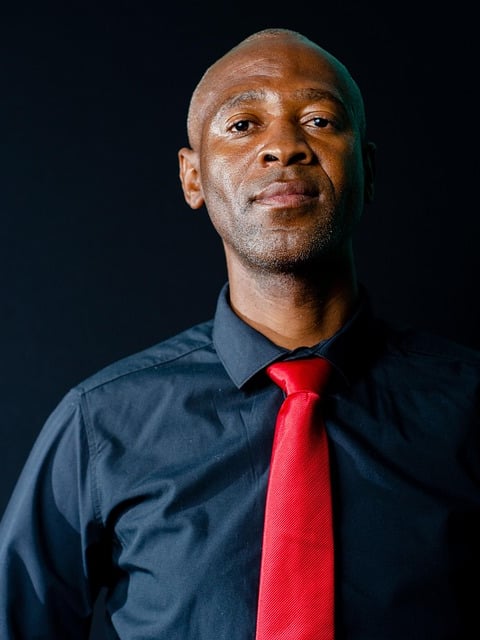
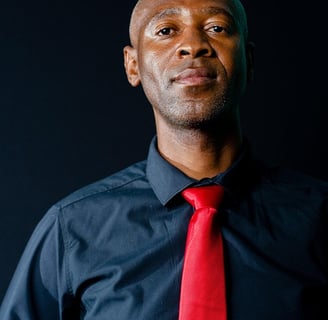
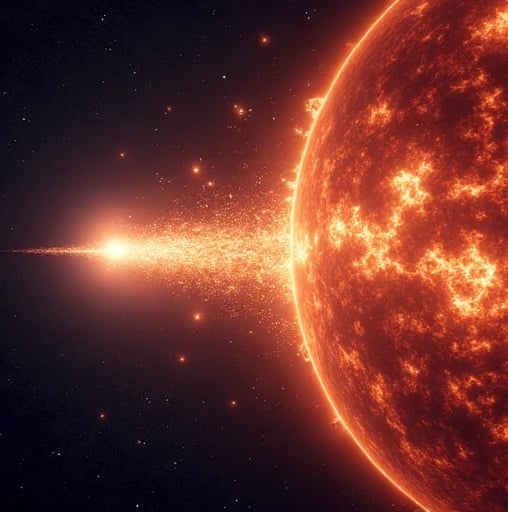

Solar Monitoring
Developing advanced anomaly detection for solar wind analysis.
Anomaly Detection
Integrating models for real-time solar wind monitoring.
Data Analysis
Tools for detecting anomalies in solar wind characteristics.
My past research has focused on the innovative field of applying solar physics principles to AI anomaly detection system design. In "AI Anomaly Detection through Solar Wind Analysis" (published in Nature Machine Intelligence, 2022), I first proposed a framework for applying solar wind particle flow analysis to AI anomaly detection. Another work, "Real-time Anomaly Recognition in AI: Lessons from Space Weather Monitoring" (NeurIPS 2022), deeply explored implications of space weather monitoring for AI anomaly recognition mechanisms. I also led research on "Adaptive Anomaly Detection through Solar Physics Principles" (ICLR 2023), which developed an adaptive anomaly detection strategy based on solar physics. The recent "From Solar Wind to AI Anomalies: A Systematic Approach" (ICML 2023) systematically analyzed the application of solar physics principles in AI anomaly detection.